The Future of Education: How AI is Transforming Personalized Learning
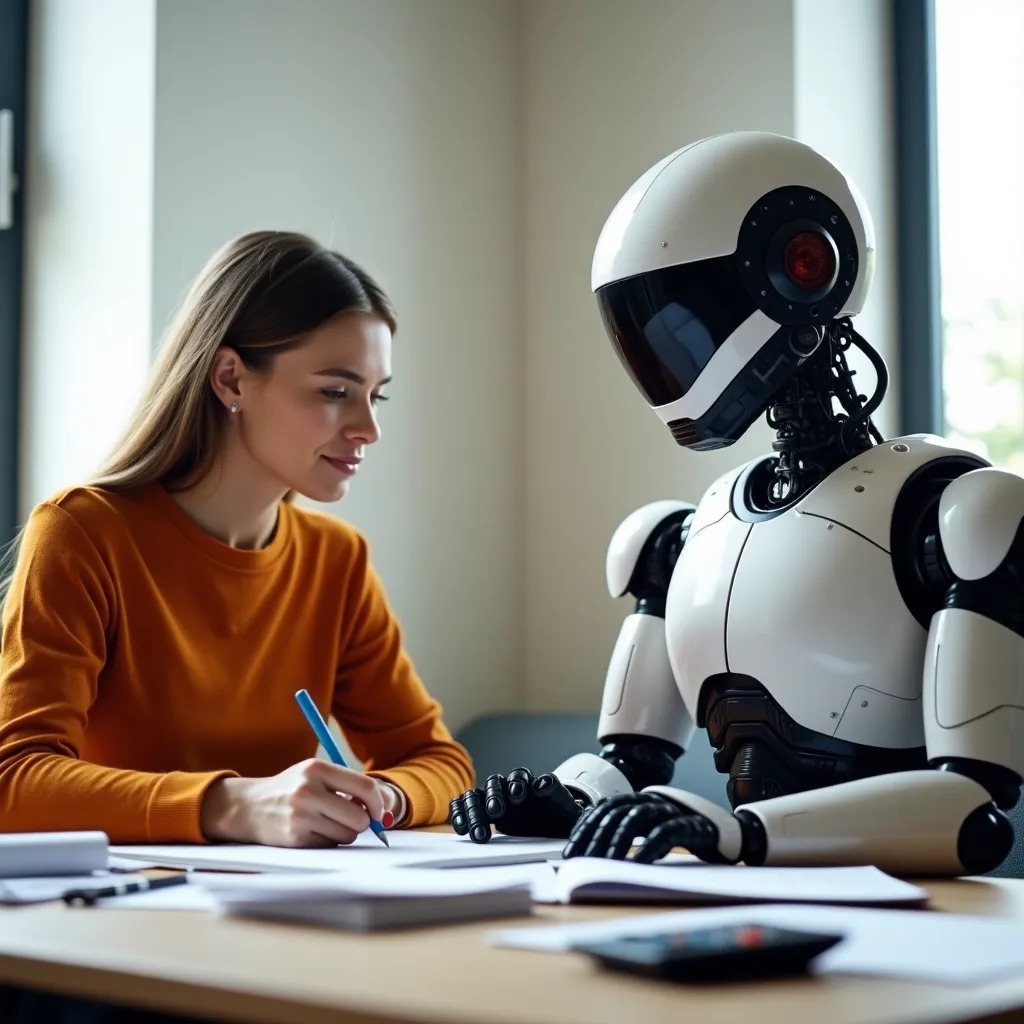
A brief history of AI technology
The idea of "artificial intelligence" goes back thousands of years, to ancient philosophers considering questions of life and death. In ancient times, inventors made things called "automatons" which were mechanical and moved independently of human intervention. The word "automaton" comes from ancient Greek, and means "acting of one's own will." One of the earliest records of an automaton comes from 400 BCE and refers to a mechanical pigeon created by a friend of the philosopher Plato. Many years later, one of the most famous automatons was created by Leonardo da Vinci around the year 1495.
So while the idea of a machine being able to function on its own is ancient, for the purposes of this article, we’re going to focus on the 20th century, when engineers and scientists began to make strides toward our modern-day AI.
1900-1950 In the early 1900s, there was a lot of media created that centered around the idea of artificial humans. So much so that scientists of all sorts started asking the question: is it possible to create an artificial brain? Some creators even made some versions of what we now call "robots" (and the word was coined in a Czech play in 1921) though most of them were relatively simple. These were steam-powered for the most part, and some could make facial expressions and even walk.
1921: Czech playwright Karel Čapek released a science fiction play "Rossum's Universal Robots" which introduced the idea of "artificial people" which he named robots. This was the first known use of the word.
1929: Japanese professor Makoto Nishimura built the first Japanese robot, named Gakutensoku.
1949: Computer scientist Edmund Callis Berkley published the book "Giant Brains, or Machines that Think" which compared the newer models of computers to human brains.
Birth of AI: 1950-1956 This range of time was when the interest in AI really came to a head. Alan Turing published his work "Computer Machinery and Intelligence" which eventually became The Turing Test, which experts used to measure computer intelligence. The term "artificial intelligence" was coined and came into popular use.
1950: Alan Turing published "Computer Machinery and Intelligence" which proposed a test of machine intelligence called The Imitation Game.
1952: A computer scientist named Arthur Samuel developed a program to play checkers, which is the first to ever learn the game independently.
1955: John McCarthy held a workshop at Dartmouth on "artificial intelligence" which is the first use of the word, and how it came into popular usage.
AI maturation: 1957-1979 The time between when the phrase "artificial intelligence" was created, and the 1980s was a period of both rapid growth and struggle for AI research. The late 1950s through the 1960s was a time of creation. From programming languages that are still in use to this day to books and films that explored the idea of robots, AI became a mainstream idea quickly.
The 1970s showed similar improvements, such as the first anthropomorphic robot being built in Japan, to the first example of an autonomous vehicle being built by an engineering grad student. However, it was also a time of struggle for AI research, as the U.S. government showed little interest in continuing to fund AI research.
Notable dates include:
1958: John McCarthy created LISP (acronym for List Processing), the first programming language for AI research, which is still in popular use to this day.
1959: Arthur Samuel created the term "machine learning" when doing a speech about teaching machines to play chess better than the humans who programmed them.
1961: The first industrial robot Unimate started working on an assembly line at General Motors in New Jersey, tasked with transporting die casings and welding parts on cars (which was deemed too dangerous for humans).
1965: Edward Feigenbaum and Joshua Lederberg created the first "expert system" which was a form of AI programmed to replicate the thinking and decision-making abilities of human experts.
1966: Joseph Weizenbaum created the first "chatterbot" (later shortened to chatbot), ELIZA, a mock psychotherapist, that used natural language processing (NLP) to converse with humans.1968: Soviet mathematician Alexey Ivakhnenko published "Group Method of Data Handling" in the journal "Avtomatika," which proposed a new approach to AI that would later become what we now know as "Deep Learning."
1973: An applied mathematician named James Lighthill gave a report to the British Science Council, underlining that strides were not as impressive as those that had been promised by scientists, which led to much-reduced support and funding for AI research from the British government.
1979: James L. Adams created The Standford Cart in 1961, which became one of the first examples of an autonomous vehicle. In '79, it successfully navigated a room full of chairs without human interference.
1979: The American Association of Artificial Intelligence which is now known as the Association for the Advancement of Artificial Intelligence (AAAI) was founded.
AI boom: 1980-1987 Most of the 1980s showed a period of rapid growth and interest in AI, now labeled as the "AI boom." This came from both breakthroughs in research, and additional government funding to support the researchers. Deep Learning techniques and the use of Expert System became more popular, both of which allowed computers to learn from their mistakes and make independent decisions.
Notable dates in this time period include:
1980: First conference of the AAAI was held at Stanford.
1980: The first expert system came into the commercial market, known as XCON (expert configurer). It was designed to assist in the ordering of computer systems by automatically picking components based on the customer's needs.
1981: The Japanese government allocated 2 billion dollars in today's money) to the Fifth Generation Computer project. Their aim was to create computers that could translate, converse in human language, and express reasoning on a human level.
1984: The AAAI warns of an incoming "AI Winter" where funding and interest would decrease, and make research significantly more difficult.
1985: An autonomous drawing program known as AARON is demonstrated at the AAAI conference.
1986: Ernst Dickmann and his team at Bundeswehr University of Munich created and demonstrated the first driverless car (or robot car). It could drive up to 55 mph on roads that didn't have other obstacles or human drivers.
1987: Commercial launch of Alacrity by Alactrious Inc. Alacrity was the first strategy managerial advisory system, and used a complex expert system with 3,000+ rules.
AI winter: 1987-1993 As the AAAI warned, an AI Winter came. The term describes a period of low consumer, public, and private interest in AI which leads to decreased research funding, which, in turn, leads to few breakthroughs. Both private investors and the government lost interest in AI and halted their funding due to high cost versus seemingly low return. This AI Winter came about because of some setbacks in the machine market and expert systems, including the end of the Fifth Generation project, cutbacks in strategic computing initiatives, and a slowdown in the deployment of expert systems.
Notable dates include:
1987: The market for specialized LISP-based hardware collapsed due to cheaper and more accessible competitors that could run LISP software, including those offered by IBM and Apple. This caused many specialized LISP companies to fail as the technology was now easily accessible.
1988: A computer programmer named Rollo Carpenter invented the chatbot Jabberwacky, which he programmed to provide interesting and entertaining conversation to humans.
AI agents: 1993-2011 Despite the lack of funding during the AI Winter, the early 90s showed some impressive strides forward in AI research, including the introduction of the first AI system that could beat a reigning world champion chess player. This era also introduced AI into everyday life via innovations such as the first Roomba and the first commercially-available speech recognition software on Windows computers.
The surge in interest was followed by a surge in funding for research, which allowed even more progress to be made.
Notable dates include:
1997: Deep Blue (developed by IBM) beat the world chess champion, Gary Kasparov, in a highly-publicized match, becoming the first program to beat a human chess champion.
1997: Windows released a speech recognition software (developed by Dragon Systems).
2000: Professor Cynthia Breazeal developed the first robot that could simulate human emotions with its face,which included eyes, eyebrows, ears, and a mouth. It was called Kismet.
2002: The first Roomba was released.
2003: Nasa landed two rovers onto Mars (Spirit and Opportunity) and they navigated the surface of the planet without human intervention.
2006: Companies such as Twitter, Facebook, and Netflix started utilizing AI as a part of their advertising and user experience (UX) algorithms.
2010: Microsoft launched the Xbox 360 Kinect, the first gaming hardware designed to track body movement and translate it into gaming directions.
2011: An NLP computer programmed to answer questions named Watson (created by IBM) won Jeopardy against two former champions in a televised game.
2011: Apple released Siri, the first popular virtual assistant.
Artificial General Intelligence: 2012-present That brings us to the most recent developments in AI, up to the present day. We’ve seen a surge in common-use AI tools, such as virtual assistants, search engines, etc. This time period also popularized Deep Learning and Big Data..
Notable dates include:
2012: Two researchers from Google (Jeff Dean and Andrew Ng) trained a neural network to recognize cats by showing it unlabeled images and no background information.
2015: Elon Musk, Stephen Hawking, and Steve Wozniak (and over 3,000 others) signed an open letter to the worlds’ government systems banning the development of (and later, use of) autonomous weapons for purposes of war.
2016: Hanson Robotics created a humanoid robot named Sophia, who became known as the first "robot citizen" and was the first robot created with a realistic human appearance and the ability to see and replicate emotions, as well as to communicate.
2017: Facebook programmed two AI chatbots to converse and learn how to negotiate, but as they went back and forth they ended up forgoing English and developing their own language, completely autonomously.
2018: A Chinese tech group called Alibaba's language-processing AI beat human intellect on a Stanford reading and comprehension test.
2019: Google's AlphaStar reached Grandmaster on the video game StarCraft 2, outperforming all but .2% of human players.
2020: OpenAI started beta testing GPT-3, a model that uses Deep Learning to create code, poetry, and other such language and writing tasks. While not the first of its kind, it is the first that creates content almost indistinguishable from those created by humans.
2021: OpenAI developed DALL-E, which can process and understand images enough to produce accurate captions, moving AI one step closer to understanding the visual world.
Overview of AI’s burgeoning role in modern education systems
The integration of AI in education has opened new avenues for personalised, efficient, and accessible learning experiences. AI-powered tutoring systems are at the forefront of this transformation, offering various tools and platforms that cater to the unique needs of each student. This blog explores how AI can be effectively utilised in education, the implementation strategies, ethical considerations, benefits, challenges, and practical tips for students.
AI-Powered Tutoring Systems
Adaptive Learning Platforms
Functionality: Adaptive learning platforms adjust the difficulty and type of content based on a student’s performance and learning pace, providing a tailored learning experience. By continuously analysing a student’s interactions and progress, these platforms can dynamically modify the instructional material to optimise learning outcomes.
DreamBox: An intelligent math learning system that adapts lessons to individual student needs, ensuring each student receives instruction at the right level of difficulty and pace to maximise understanding and retention.
Knewton: This platform utilises advanced algorithms to personalise educational content for students. Knewton’s technology analyses millions of data points to determine how students learn best, providing tailored recommendations that help improve comprehension and retention.
Smart Sparrow: Offers adaptive eLearning solutions that respond to student interactions in real-time, providing personalised feedback and guidance to enhance learning experiences.
Virtual Tutors and Chatbots
Functionality: AI tutors and chatbots can provide instant answers to student queries, offer explanations for complex topics, and guide students through problems. These systems can simulate one-on-one tutoring experiences, making personalised assistance more accessible.
IBM Watson Tutor: Uses AI to provide personalised tutoring and academic support. Watson can understand natural language questions and provide detailed, contextually appropriate answers and explanations.
Duolingo: Employs AI-driven language practice to enhance language learning experiences. Duolingo’s AI tailors lessons to the user’s learning style and pace, providing instant feedback and correction.
Replika: A chatbot that aids in conversational practice and emotional support, helping users improve their language skills through natural, engaging conversations.
Feedback and Assessment
Functionality: AI can deliver personalised feedback on assignments, quizzes, and exams, highlighting areas for improvement. These systems can provide detailed insights into student performance, helping educators identify learning gaps and address them promptly.
Grammarly: Provides real-time writing feedback, focusing on grammar, style, and tone. Grammarly’s AI analyses writing for correctness, clarity, engagement, and delivery, offering suggestions to enhance writing skills.
Gradescope: Automates grading and provides detailed feedback to help students improve. Gradescope’s AI can grade a wide range of assignment types, from handwritten work to programming assignments, ensuring consistency and efficiency in grading.
Supplemental Learning Resources
Functionality: AI can recommend additional resources such as videos, articles, and exercises based on the student’s learning history and needs. This personalised approach ensures students receive relevant and helpful supplementary materials to reinforce their learning.
Khan Academy: Uses AI to suggest videos and exercises tailored to the student’s progress, helping them build on their existing knowledge and address areas of weakness.
Coursera: Recommends personalised course suggestions to enhance learning paths. Coursera’s AI considers the user’s interests, previous course completions, and learning goals to suggest relevant courses and materials.
Tools for Enhanced Understanding (Not for Copy-Paste)
Functionality: These tools are designed to foster deeper understanding rather than providing direct answers or content for copy-pasting. They support critical thinking and active learning through interactive and engaging educational experiences.
Socratic by Google: Uses AI to help students understand their homework questions. Students can take a photo of their question, and Socratic provides step-by-step explanations, related resources, and detailed walkthroughs.
Wolfram Alpha: An AI-powered computational engine that helps students solve mathematical problems, understand complex concepts, and explore scientific queries through detailed explanations and visualisations.
Quizlet: Offers AI-powered study tools, including flashcards and practice tests, that adapt to the student’s learning progress. Quizlet’s AI helps identify areas where students need more practice and reinforces their knowledge through repeated exposure.
Edmodo: A social learning platform that uses AI to recommend resources and connect students with peers and educators for collaborative learning. In addition, Edmodo’s AI helps facilitate discussions, provide feedback, and promote active engagement with the material.
Zearn: An AI-driven math learning platform that provides interactive lessons and practice problems. Zearn’s AI adapts to each student’s progress, offering hints, step-by-step solutions, and alternative explanations to ensure comprehension.
Implementation Strategies
Integration with Curriculum: Ensure that AI tools align with the existing curriculum and complement traditional teaching methods rather than replace them. This integration helps maintain a cohesive educational experience while enhancing it with personalised support.
Teacher Involvement: Teachers should guide the use of AI tools, helping students understand their effective and ethical usage. Educators play a crucial role in interpreting AI-generated insights and integrating them into the broader teaching strategy.
Training and Support: Provide comprehensive training for both students and educators on how to use AI tutoring systems to maximise their benefits. Ongoing support and professional development ensure that all users can effectively leverage these tools.
Ethical Considerations
Data Privacy: Protect student data by ensuring AI tools comply with data protection regulations such as GDPR. Secure data handling practices and transparent data use policies are essential to maintaining trust.
Bias and Fairness: Monitor AI systems for biases that may disadvantage certain groups of students. Regularly update and audit AI algorithms to ensure fairness. Addressing bias proactively helps create an equitable learning environment.
Transparency: Clearly explain to students how AI tools work and how their data is used. Transparency builds trust and encourages responsible use. Providing insights into AI decision-making processes can help demystify the technology and promote informed usage.
Benefits of AI-Powered Tutoring
Personalisation: AI tailors learning experiences to meet the unique needs of each student, enhancing engagement and effectiveness. Personalised learning paths help students progress at their own pace, addressing individual strengths and weaknesses.
Accessibility: AI tutoring systems provide support anytime, anywhere, making education more accessible. This flexibility is particularly beneficial for students with varying schedules and learning environments.
Efficiency: AI handles repetitive tasks, allowing educators to focus on more complex teaching activities. By automating grading, feedback, and administrative tasks, teachers can dedicate more time to personalised instruction and student support.
Challenges and Limitations
Over-reliance on Technology: Students may become too dependent on AI tools, potentially hindering the development of independent problem-solving skills. Balancing AI use with traditional learning methods is essential to foster critical thinking and self-reliance.
Quality of Content: Ensuring that AI provides accurate and high-quality content is crucial. Regular updates and human oversight are necessary to maintain the integrity of educational materials. Educators should continuously review AI-generated content to ensure its relevance and accuracy.
Digital Divide: Not all students have equal access to technology. Addressing this divide is crucial to ensure that AI benefits all students. Providing equitable access to devices and internet connectivity is essential to leveraging AI in education.
Practical Tips for Students
Empowering Learning: How AI is Revolutionizing Math and Science Education
Introduction to AI in Education
The journey of artificial intelligence (AI) through the ages—from ancient automata to today’s sophisticated algorithms—illuminates humanity's enduring fascination with simulating life and intelligence. The 20th century marked an era synonymous with rapid advancements in AI, culminating in what we know today as the digital revolution. AI’s integration into educational systems is not just transformative but also heralds a new era of personalized learning, especially in core subjects like math and science.
AI Applications Revolutionizing Learning
Personalized Learning Paths: Adapting to Student Needs in Math and Science
In subjects that often have a steep learning curve such as math and science, AI's ability to tailor content to the pace and proficiency of each student is invaluable. Platforms like DreamBox provide math learning experiences that automatically adjust to students' responses, making math more accessible and engaging. This adaptive learning approach ensures students are neither bored with content that's too easy nor overwhelmed by that which is too demanding.
AI-Driven Virtual Tutors: Enhancing Understanding and Engagement
Virtual tutors powered by AI, such as IBM’s Watson Tutor, reshape how students interact with educational content. These tutors provide immediate, customized assistance, be it walking a student through a complex algebra problem or explaining a scientific concept in depth. The result is a learning process that is more engaging and attuned to individual learning styles.
Multifaceted Benefits of AI in Educational Settings
How Students are Achieving More with Personalized AI Support
With AI, the promise of personalized education is becoming a reality. Students in math and science subjects can benefit from AI that pinpoints their individual challenges and accelerates their progress by continuously adapting to their learning needs.
Empowering Teachers with AI Tools: A Closer Look at Efficiency and Effectiveness
Teachers are also beneficiaries of AI's capabilities, as these tools take over time-consuming tasks such as grading and assessments. This automation allows teachers to dedicate more attention to creative and interactive teaching practices, thus increasing overall classroom productivity.
The Role of AI in Involving Parents in the Educational Process
AI tools also bridge communication between parents and educational systems, providing real-time updates on student progress and areas needing attention. This involvement encourages a supportive home learning environment, crucial for student success, especially in demanding subjects like math and science.
Forecasting AI's Future in Education: 2023-2033
Innovations on the Horizon: What's Next for AI in Learning?
Looking forward, AI is poised to introduce more sophisticated virtual reality (VR) and augmented reality (AR) environments that can simulate real-world science labs or math workshops, offering hands-on experience without the logistical limitations.
AI’s Potential to Transform Educational Assessment and Accreditation
One of the most significant shifts AI may bring is in assessment systems. AI’s potential to analyze vast amounts of data about a student's learning journey could lead to more nuanced and comprehensive assessments than traditional methods provide.
Challenges and Opportunities: Balancing AI Advancements with Ethical Considerations
Navigating Data Privacy and Security in AI Applications
As educational institutions adopt more AI tools, the importance of securing student data becomes paramount. Adequate measures and regulations need to ensure that students' personal and academic information is protected against any misuse.
The Opportunity to Narrow Educational Gaps: AI as a Leveling Tool in Diverse Societies
AI holds the promise of democratizing education—providing high-quality learning experiences to remote areas and underserved communities. However, it is crucial to address and close the digital divide to ensure that these transformative tools benefit everyone equally.
The dynamic between AI and education is unfolding rapidly, promising a future where learning is not only personalized but also more accessible and engaging. As we embrace this new era, the sustained collaboration between technology experts and educators will be critical to crafting educational experiences that benefit all students, preparing them for a future where AI will be a fundamental aspect of everyday life.
Table of Contents
- The Future of Education: How AI is Transforming Personalized Learning
- A brief history of AI technology
- Overview of AI’s burgeoning role in modern education systems
- Personalized Learning Paths: Adapting to Student Needs in Math and Science
- AI-Driven Virtual Tutors: Enhancing Understanding and Engagement
- How Students are Achieving More with Personalized AI Support
- Empowering Teachers with AI Tools: A Closer Look at Efficiency and Effectiveness
- The Role of AI in Involving Parents in the Educational Process
- Innovations on the Horizon: What's Next for AI in Learning?
- AI’s Potential to Transform Educational Assessment and Accreditation
- Navigating Data Privacy and Security in AI Applications
- The Opportunity to Narrow Educational Gaps: AI as a Leveling Tool in Diverse Societies
Related Posts
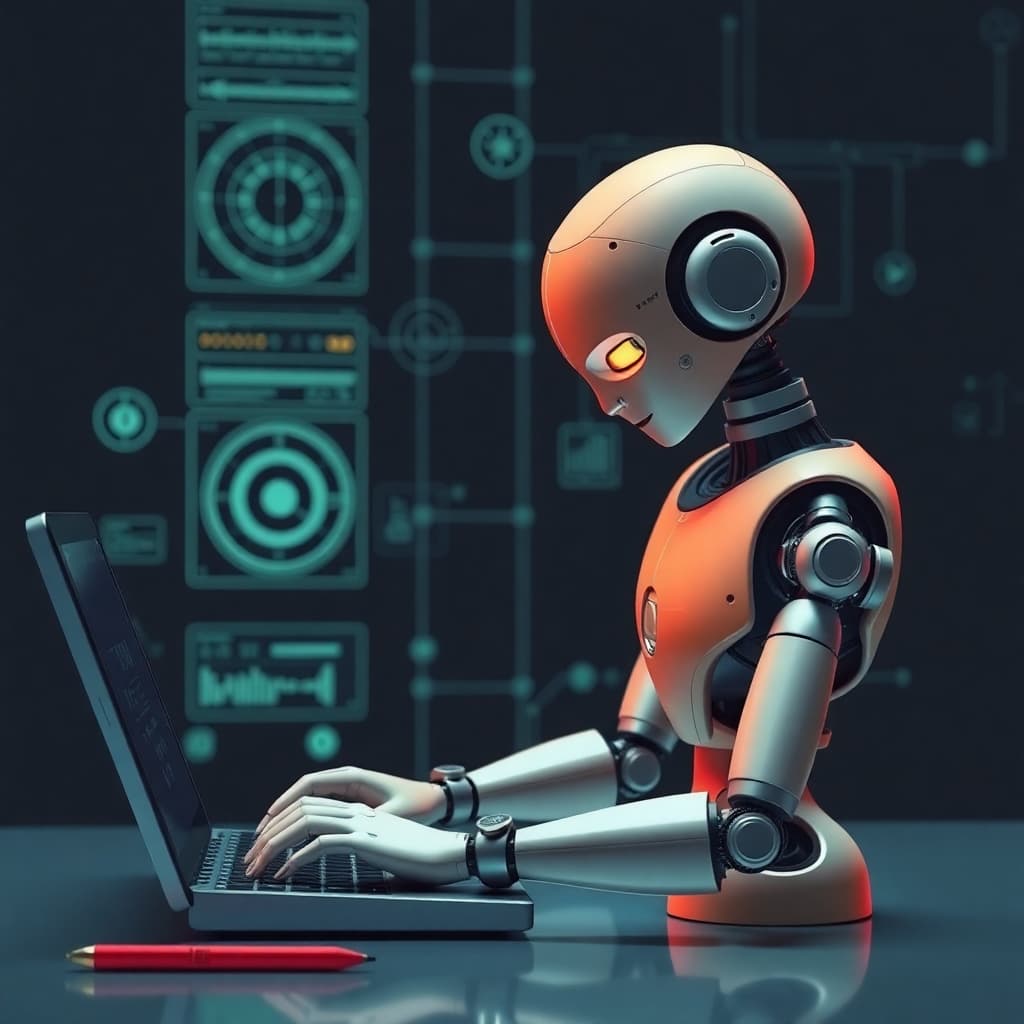
Discover how AI is transforming educational assessment, moving beyond traditional testing to provide more accurate, comprehensive, and fair evaluations of student learning.
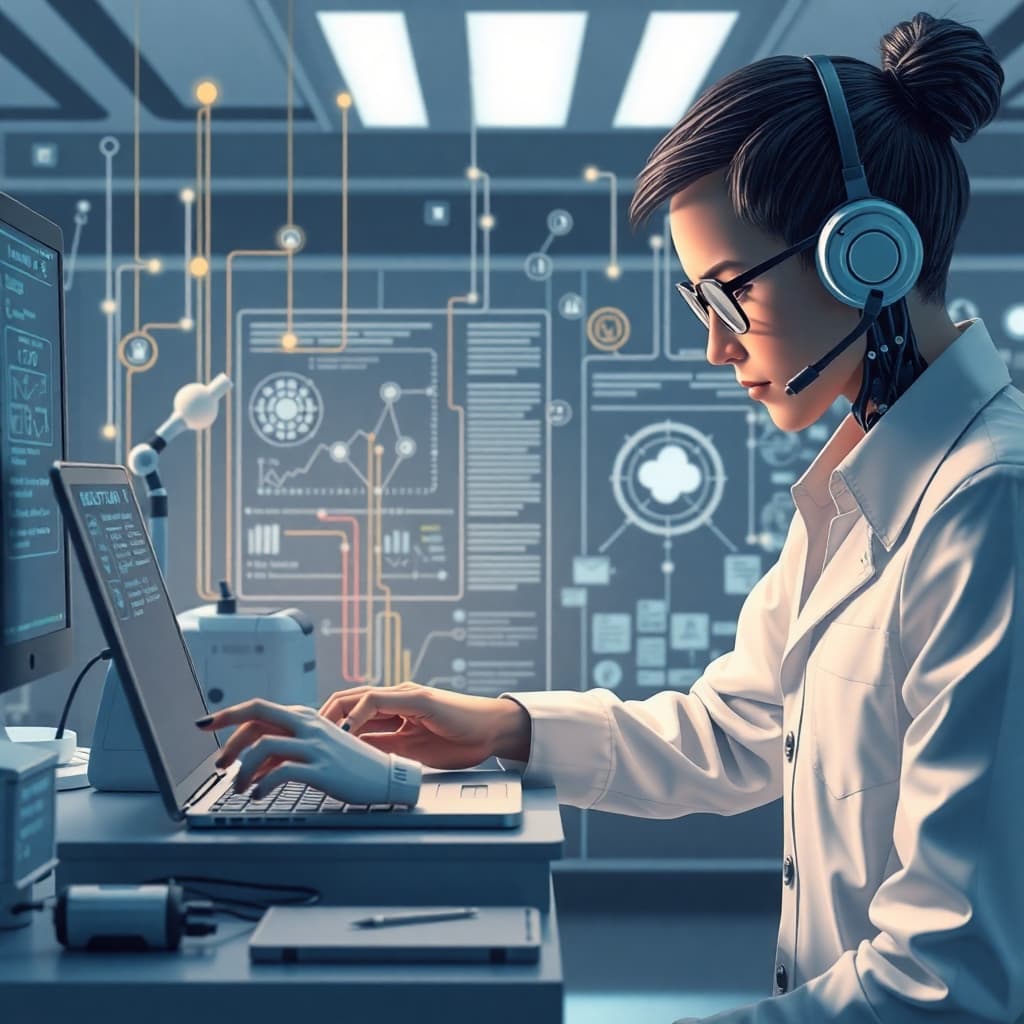
Explore how AI is enhancing collaborative learning experiences, preparing students for the future of work through innovative group projects and team-based problem-solving.
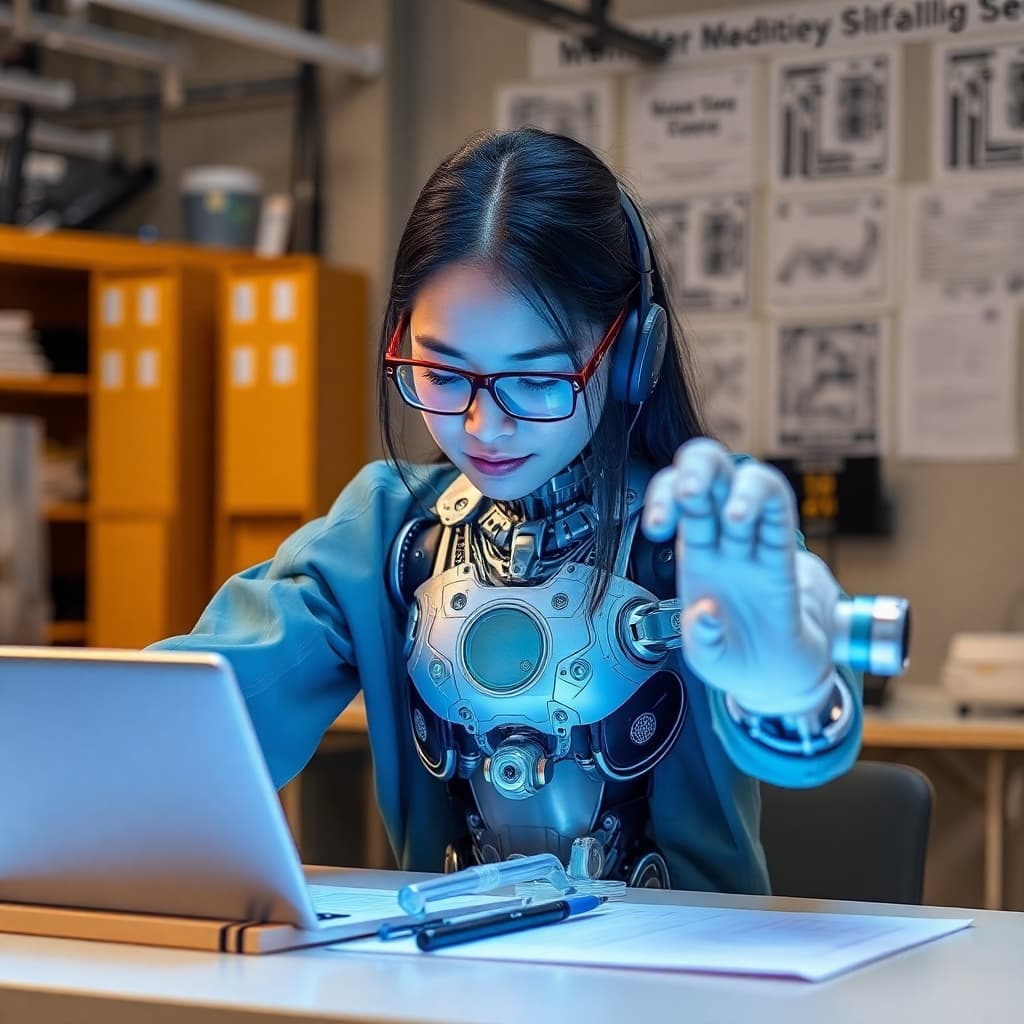
Explore how AI is being used to develop emotional intelligence in students, creating more empathetic, self-aware, and socially skilled learners for the 21st century.
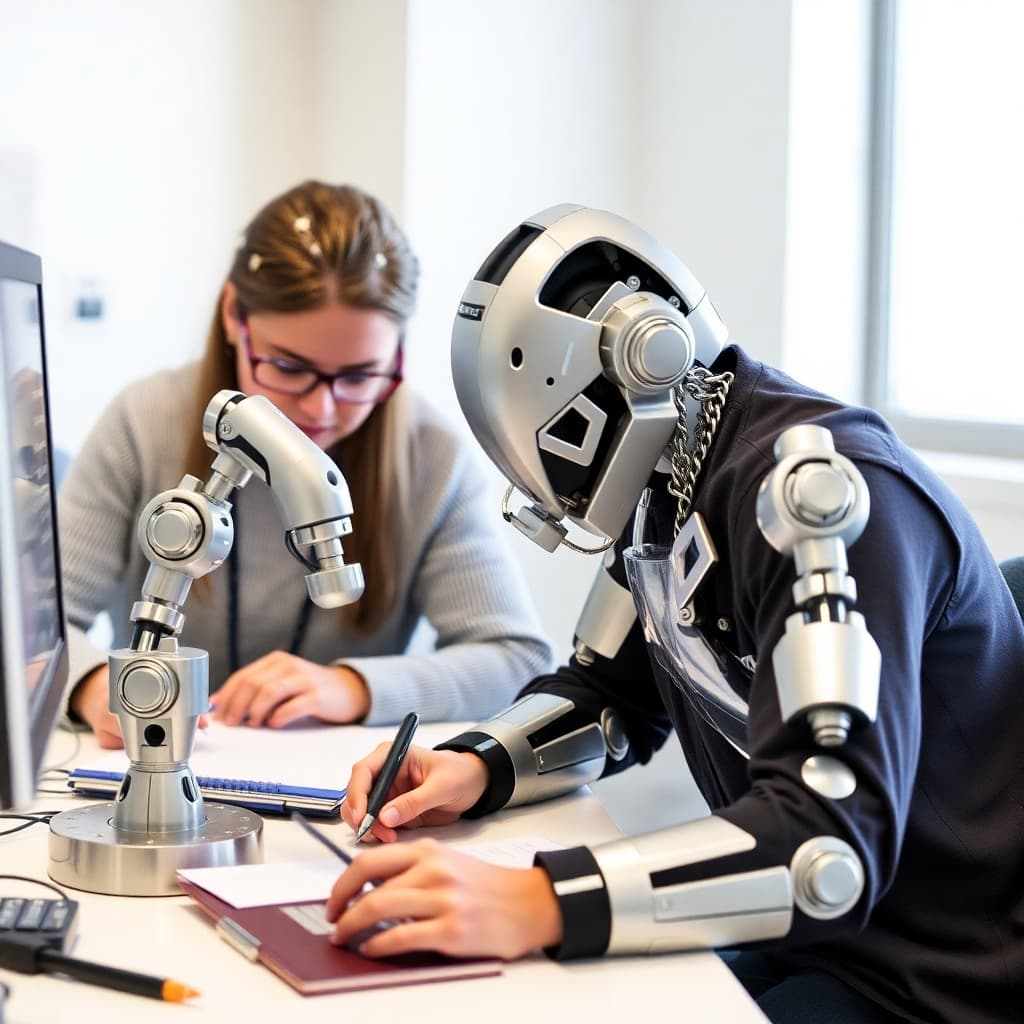
Discover how AI is breaking down barriers in education, providing personalized support for students with diverse needs and learning differences.
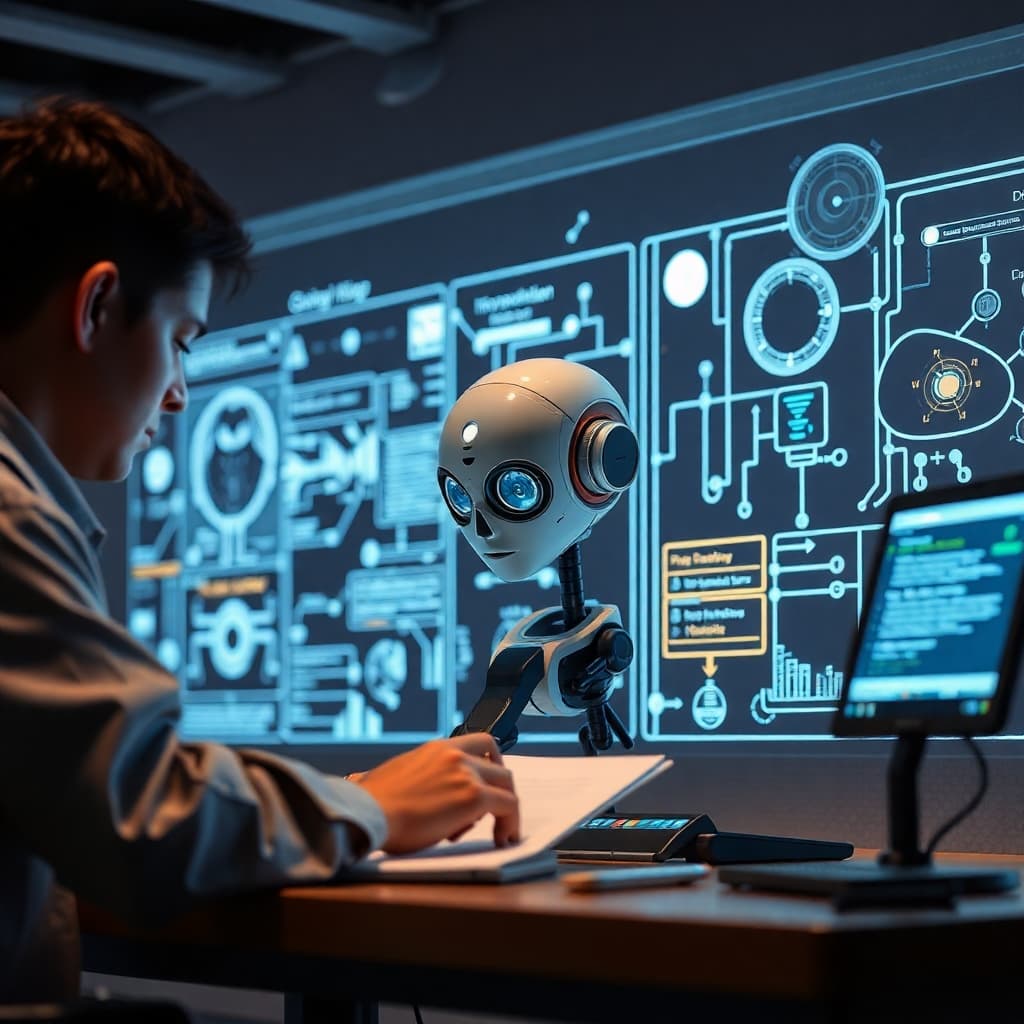
Discover how AI is transforming language education, making it more accessible, personalized, and effective for learners worldwide.
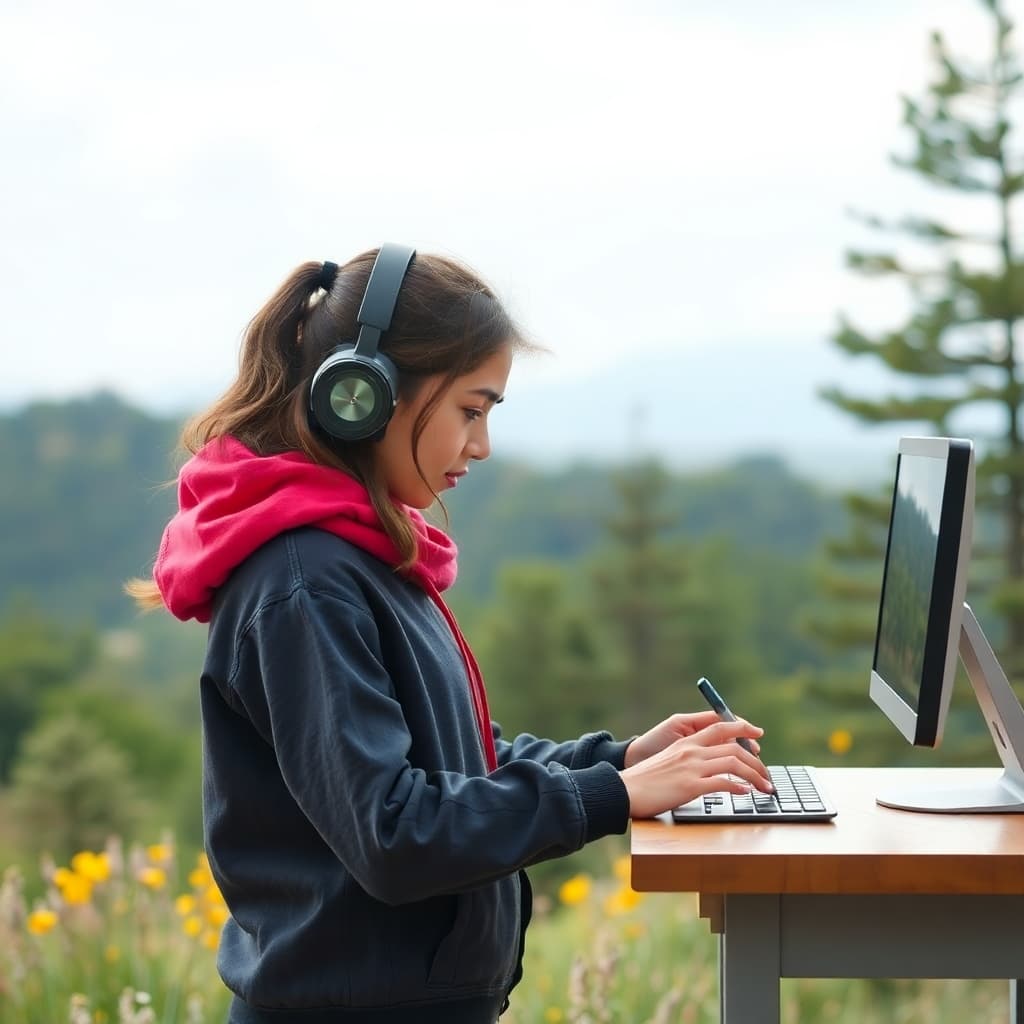
Discover how artificial intelligence is revolutionizing study methods, helping students learn more effectively and retain information longer.